The Power of Product Testing with Synthetic Data
Read more about generating and evaluating high-quality synthetic data and explore how synthetic data can be applied specifically to product testing.
Synthetic data is revolutionizing industries from healthcare to financial services to automotive, by enabling simulations and data augmentation. At Ipsos, we believe synthetic data opens up brand new possibilities for market research, particularly in the area of product testing. However, many businesses remain uncertain about the quality of synthetic data or how to evaluate it.
In this paper, we provide recommendations for generating and evaluating high-quality synthetic data and explore how synthetic data can be applied specifically to product testing.
Generating and evaluating synthetic data
To generate synthetic data that effectively mimics real-world data, an artificial intelligence (AI) model must first be trained on relevant, real-world data. As discussed in our first paper in the Humanizing AI series, AIs are simply algorithms; they have no intelligence of their own, until they are trained. It is through learning from training data that AIs acquire the intelligence we associate with them.
The evaluation process is also straightforward. Synthetic numerical data should, at minimum, mirror real-world data on common statistical measures. The closer synthetic data is to human data, the less risk we assume when using it, but there is always some risk because synthetic data can never perfectly mimic real-world data in every aspect.
Approaches to generating synthetic data fall into two categories: LLMs (Large Language Models) and non-LLMs, distinguished by their textual and numerical nature, respectively. We explore both approaches in this paper.
The product experience is inherently human
How humans react to products, or life in general, is not captured solely in the brain as factual or semantic knowledge, our bodies and sensory experiences play a significant role, too.
This paper presents the findings from our two research streams carried out aiming to establish the minimum number of human respondents needed to test products alongside synthetic data to ensure viable results. To discover the key findings from the research, you can also download our useful infographic.
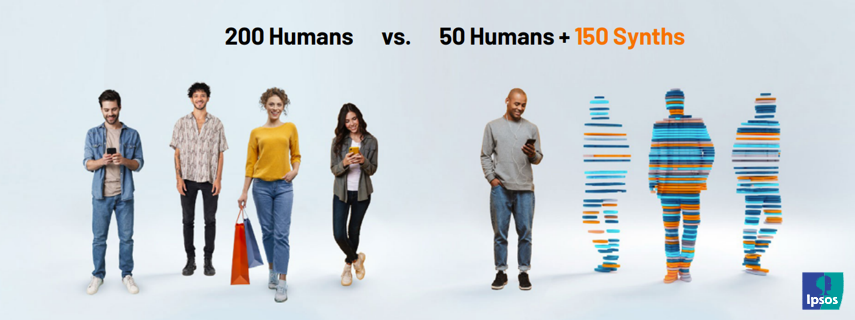
If an AI has not been trained on real-world data that is relevant to your business, it will not be able to generate synthetic data that shares the same properties as real-world data. It’s as simple as that!
Key takeaways:
1. Synthetic data will never be human.
AI alone can never echo our product experiences, which combine the five senses, emotions, expectations, and context. Therefore, our goal is to augment human input with synthetic data, not replace it.
2. Accuracy hinges on the training data.
The value of synthetic data is not binary (good or bad); the accuracy of synthetic data depends on many factors including the differences in the data we are trying to replicate, and the representativeness of the real-world data we are training an AI to learn from. The use of synthetic data should be strategic, considering the associated risks and benefits.
3. When accurate, it can power product testing.
Synthetic data can boost market research agility, making it ideal for resource-intensive areas like product testing - reducing costs, saving time, with additional benefits for detailed sub-group analyses.